Generative AI: What Is It? Why Is It Important? and How Does It Operate?
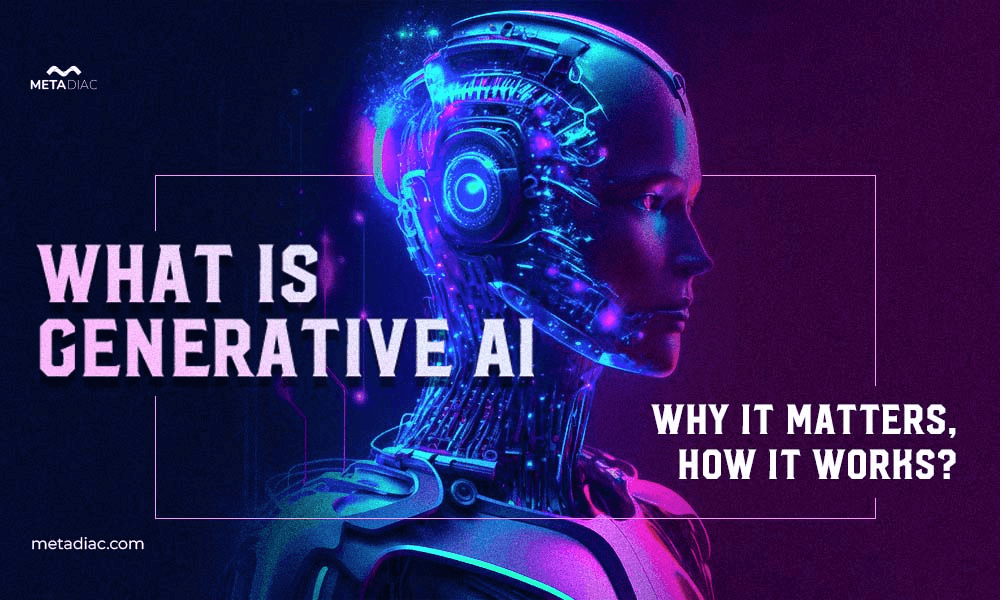
Have you ever thought about how AI can create art, music, or even write stories just like humans do? Well, that's what Generative AI is all about. It's a fascinating area of artificial intelligence that focuses on creating new content, whether it’s images, text, or sounds, from scratch. Imagine a machine that can compose a beautiful symphony or generate a detailed painting based on a few prompts. Amazing, right?
When ChatGPT was at its extent, people were flying around with colors as this advancement in AI technology simplified their business lives. This technology has seen a swift rise in recent years, with applications ranging from art and music to text generation and software development.
This blog will walk you through why this technology is capturing everyone’s attention and why it’s set to be a game-changer in our digital world. So, stick around and read on to discover the popularity behind generative AI!
What Is Generative AI?
Generative AI, a revolutionary branch of artificial intelligence, is transforming the way we create and interact with digital content. Originating from advancements in machine learning and neural networks, generative AI involves algorithms that can produce new, original data based on the patterns and structures of the input data they were trained on.
To put its growth into perspective, the global generative AI market was valued at approximately $6.3 billion in 2020 and is projected to reach $200 billion by 2030, according to a report by MarketsandMarkets. In 2023 alone, investment in AI startups surged by 40%, with a significant portion directed toward generative AI technologies.
A striking real-life example of generative AI in action is the work of OpenAI's GPT-3. This powerful language model can generate human-like text based on a few prompts. It has been used to write articles, create poetry, and even generate code.
DALL-E, another project by OpenAI, showcases the power of generative AI in visual creativity. DALL-E can generate images from textual descriptions, such as "a two-story pink house shaped like a shoe." This ability to turn words into images has numerous applications, from helping designers visualize concepts to assisting in education and entertainment.
Why does Generative AI play a Significant Role?
Generative AI has gained immense popularity recently, and here's why:
AIVA (Artificial Intelligence Virtual Artist): AIVA is a generative AI that composes classical music. It has been used to create soundtracks for movies, video games, and advertisements. This tool allows composers to experiment with new musical ideas and produce high-quality music faster than traditional methods.
GPT-4: Also developed by OpenAI, GPT-4 can write articles, generate dialogue, and even compose poetry. Businesses use it to draft marketing content, bloggers to generate ideas, and novelists to overcome writer's block. For instance, a company might use GPT-4 to create personalized email campaigns or social media posts, saving time and enhancing engagement.
Protein Folding by DeepMind's AlphaFold: Generative AI has been used to predict the 3D structures of proteins, which is crucial for understanding diseases and developing new medicines. AlphaFold has solved some of the most complex problems in biology, helping researchers understand the molecular basis of diseases.
GANs (Generative Adversarial Networks): Companies like Zalando use GANs to design clothing by generating new fashion styles based on current trends and historical data. This not only accelerates the design process but also helps in predicting future fashion trends.
Generative AI's popularity is a result of technological advancements, its impressive capabilities, broad applications, economic benefits, accessibility, cultural impact, and ongoing research.
How Does Generative AI Work?
Generative AI works by using algorithms to create new data, such as text, images, music, or even entire virtual environments. Here's a simple breakdown of how it works:
Training Data: Generative AI models are trained on large datasets that include various examples of the type of data they need to generate. For instance, a text-generating AI would be trained on a vast amount of written content.
Learning Patterns: During training, the AI learns the underlying patterns and structures in the data. For text, this means understanding grammar, context, and style. For images, it might mean recognizing shapes, colors, and textures.
Generating New Data: Once trained, the AI can use its learned knowledge to create new content. It does this by predicting what comes next in a sequence based on the patterns it has learned. For example, given a starting sentence, a text generator can write a coherent continuation.
Feedback Loop: Some generative models use feedback mechanisms to improve their outputs. For instance, Generative Adversarial Networks (GANs) have two parts: a generator that creates data and a discriminator that evaluates it. The generator aims to produce data indistinguishable from the real data, while the discriminator tries to identify whether the data is real or generated. This process continues until the generated data is high-quality.
The Power of Generative AI Models
Generative AI comes in various forms, each designed to create different types of content or solve specific problems. Here are some common types:
Generative Adversarial Networks (GANs)
GANs consist of two neural networks: a generator and a discriminator. The generator creates new data, while the discriminator evaluates it. They work together in a feedback loop to improve the quality of the generated data.
Example: GANs can generate realistic images of people who don't exist, as seen in projects like "This Person Does Not Exist”
Variational Autoencoders (VAEs)
VAEs are used for generating data with a known distribution. They encode input data into a latent space and then decode it to generate new data.
Example: VAEs can be used to generate new handwritten digits similar to those in the MNIST dataset.
Transformer Models
Transformers, such as GPT-3, are a type of model used primarily for text generation. They use attention mechanisms to understand context and generate coherent text.
Example: GPT-3 can write essays, answer questions, and create conversational agents.
Recurrent Neural Networks (RNNs)
RNNs are designed for sequential data and can generate sequences such as text, music, or time series data.
Example: RNNs can generate new text based on an input sequence, useful in chatbots and language modeling.
Autoregressive Models
These models generate data by predicting the next value in a sequence based on previous values.
Example: Models like PixelRNN can generate images pixel by pixel.
Diffusion Models
Diffusion models generate data by reversing a gradual process of adding noise to the data. They learn to denoise data to generate new samples.
Example: Diffusion models are used in image synthesis, creating high-quality images from random noise.
Flow-Based Models
Flow-based models create data by turning a simple distribution (like a Gaussian) into a complex one using reversible functions.
Example: Flow-based models can generate realistic 3D point clouds or other complex data structures.
Style Transfer Models
These models generate new images by combining the style of one image with the content of another.
Example: Neural Style Transfer can take the style of a famous painting and apply it to a photograph.
DeepDream
DeepDream enhances patterns in images to create dream-like, surreal images by amplifying features detected by the neural network.
Example: DeepDream can create artistic interpretations of input images, making them appear fantastical.
Speech Synthesis Models
These models generate human-like speech from text input, often using neural networks trained on large datasets of spoken language.
Example: Models like WaveNet and Tacotron generate natural-sounding speech for virtual assistants and text-to-speech applications.
Generative AI continues to evolve, with new models and techniques emerging that push the boundaries of what machines can create. Each type of generative AI has its strengths and applications, contributing to advancements in various fields such as art, music, language, and beyond.
Check: AI Development Company
Generative AI: Why Does It Matter? and How Does It Benefit Us?
Generative AI offers numerous benefits across various fields, providing innovative solutions and enhancing productivity. Here are some key benefits:
Creativity and Innovation
Generative AI can produce new ideas, designs, and solutions that might not be conceived by humans. It can create art, music, and literature, inspiring creativity and opening new avenues in the arts and entertainment industries.
Automation and Efficiency
Generative AI can automate repetitive tasks, reducing the time and effort required for content creation. This leads to increased efficiency and productivity.
Personalization
Generative AI can create personalized content tailored to individual preferences, enhancing user experiences and engagement.
Enhanced Problem Solving
Generative AI can explore a wide range of solutions to complex problems, providing innovative approaches that might be overlooked by humans.
Cost Reduction
By automating tasks and processes, generative AI can significantly reduce costs in various industries.
Data Augmentation
Generative AI can create synthetic data to augment real datasets, improving the performance of machine learning models.
Improved Decision Making
Generative AI can simulate different scenarios and outcomes, helping businesses and organizations make informed decisions.
Healthcare Advancements
Generative AI can assist in creating new medical treatments, personalized medicine, and advanced diagnostic tools.
Content Creation
Generative AI can produce high-quality content for marketing, entertainment, and education, enhancing the value and diversity of available media.
Enhanced Learning and Training
Generative AI can create realistic training simulations and educational content, improving learning experiences and outcomes.
Support for Scientific Research
Generative AI can aid in scientific research by generating hypotheses, simulations, and models, accelerating the pace of discovery.
Generative AI's ability to create new and valuable content, optimize processes, and provide personalized experiences makes it a powerful tool in various domains, driving progress and innovation.
Ending Note
To wrap things up, Generative AI is a fascinating and powerful technology that's making waves in various fields. Businesses can use Generative AI to automate the creation of marketing materials, such as blog posts, social media updates, and advertisements. This saves time and ensures a consistent brand voice. By integrating Generative AI into their operations, businesses can not only streamline their processes and reduce costs but also foster innovation and enhance customer satisfaction. The transformative potential of this technology makes it an invaluable asset for businesses looking to thrive in the modern digital era. Team up with MetaDiac to explore more about our Generative AI Development Services and how we can help you achieve your business goals.